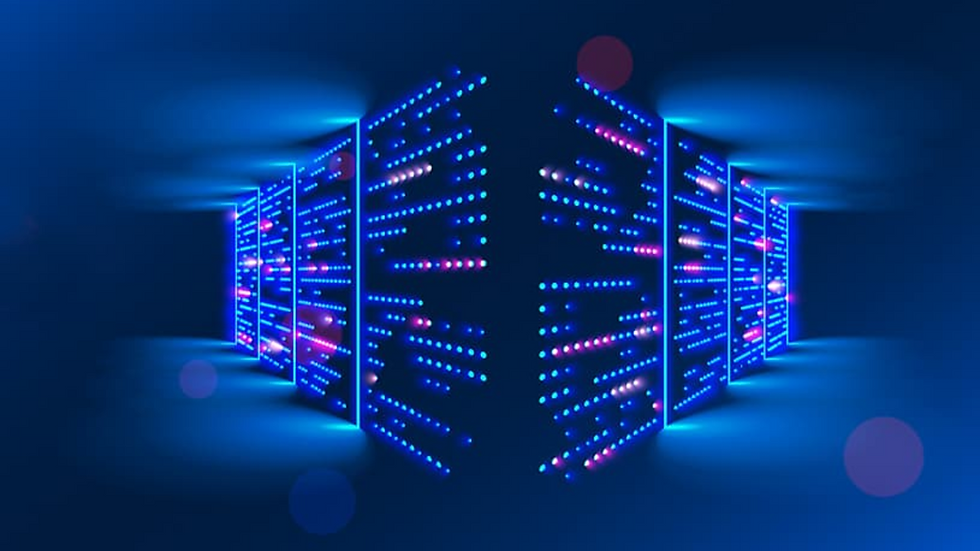
With its ability to store data from various internal and external sources, the data warehouse is considered an important element in modern business intelligence. But in what way did the data warehouse transform business intelligence? In attempting to answer this question, it is important to learn a brief history of how data warehousing has evolved.
How the need for data warehousing arose
In the days before the cloud, data was stored in physical storage solutions, evolving from data cards to magnetic storage. Then, commercial online applications came into play by the 1960s, making it possible for data to be accessed directly by computers and information to be shared between them and pave the way for online data processing. There were still challenges though, particularly finding specific data which remained difficult, and that data was not necessarily trustworthy as the information provided may be outdated or inaccurate and could not be easily verified.
Personal computer technology would eventually arise as a result of that confusion and lack of trust. For one, it lets anyone bring their computer to work and do processing when convenient. This led to personal computer software and the realization that the personal computer’s owner could store their “personal” data on their computer. With this change in work culture, it was thought that a centralized IT department might no longer be needed. Simultaneously, 4GL technology was developed based on the idea that programming and system development should be straightforward and anyone can do it. This new technology also prompted the disintegration of centralized IT departments.
4GL technology and personal computers effectively freed the end user, allowing them to take much more control of the computer system and find information quickly and efficiently. These developments quickly gained popularity in the corporate environment and are a significant step forward. However, there was still the lingering problem of incorrect, incomplete, and unverified data that needed to be effectively addressed.
This was further compounded with the rise of the internet in the 1990s as well as increased competition due to new free trade agreements, computerization, globalization, and networking. By the 2000s, many businesses discovered that with the expansion of databases and application systems, their systems had been badly integrated and that their data was inconsistent and fragmented.
It became clear that there was a need for data to be integrated to provide the critical business information needed for decision-making in a competitive, constantly changing global economy. This new reality required greater business intelligence, resulting in the need for true data warehousing.
The data warehouse of today
The data warehouses of today are typically built using relational database management systems (RDBMS), which are well-suited for data warehousing because of their efficiency in storing and querying large amounts of data.
They have also incorporated various database structures and techniques to cater to the complexities of contemporary data needs. Key among these are columnar storage systems such as Google BigQuery, Amazon Redshift, and Snowflake, which store data column-wise for faster read operations typical in analytics. Many also harness the power of Massively Parallel Processing (MPP) to distribute data tasks across multiple nodes, ensuring rapid queries on vast datasets.
Some warehouses, recognizing the need for real-time insights, adopt Hybrid Transactional/Analytical Processing (HTAP) to provide immediate insights without ETL delays. The diverse ecosystem also sees the inclusion of specialized databases, such as in-memory, time-series, and graph databases, to suit varied use cases.
Modern data warehouses can also seamlessly integrate with data lakes and natively handle semi-structured data formats like JSON. Moreover, cloud-native solutions like Snowflake, Google BigQuery, and Databricks exploit cloud elasticity for scalability benefits.
The future of data warehousing
The cloud data warehouse market is growing rapidly as more and more organizations move their data to the cloud. This growth is being driven by the benefits of cloud computing, such as scalability, flexibility, and cost-effectiveness. Furthermore, the larger players like AWS, Azure, and GCP are also offering a wide range of features and services, and they are constantly innovating.
The evolution of data warehousing in the era of business intelligence reflects the changing needs and challenges of modern organizations in making informed decision-making through data. Indeed, data warehousing solutions have evolved to become more agile, scalable, and integrated with BI tools. By embracing modern data warehousing architectures and best practices, enterprises can unlock the full potential of their data assets and gain a competitive advantage.
Comments