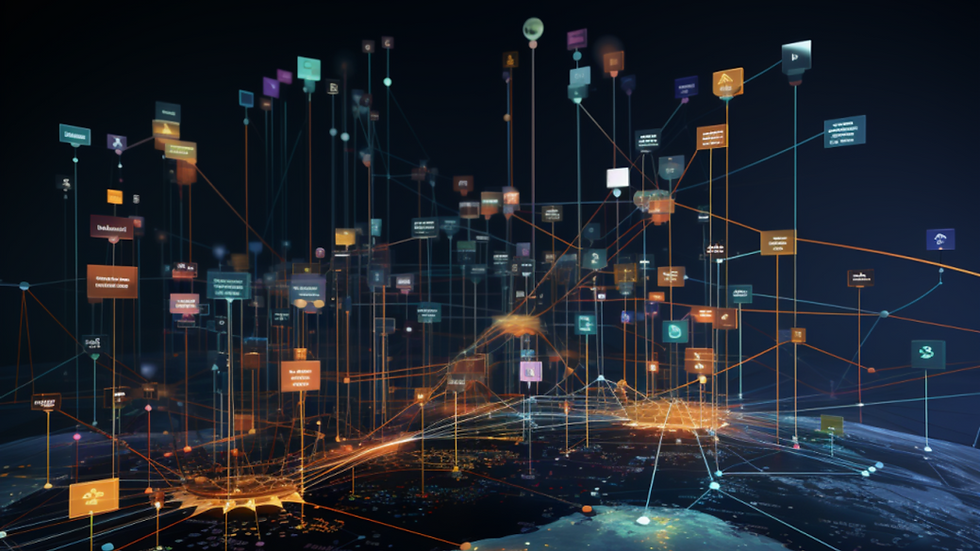
As businesses today are gathering and storing increasing amounts of data each day for their various needs, some businesses find themselves being left behind as they struggle in finding ways to make use of this increasing data.
Given the importance of data in the enterprise, it is important for businesses to able to efficiently manage and utilize their data. And the first step in achieving this goal is to understand how their data relates to their organization and to one another as a whole, as a part of a larger and more extensive data ecosystem.
To be specific, a data ecosystem not only refers to the data itself but more importantly, it refers to the programming languages, packages, algorithms, cloud-computing services, and general infrastructure an organization uses to collect, store, analyze, and leverage data.
Given the different data requirements and usage between organizations, each organization would have its own unique data ecosystem. Nevertheless, they share five key components that altogether help make data usage more efficient for the organization. These components are:
Sensing Sensing refers to the process of identifying and evaluating data sources for a particular project whether they are valuable or reliable for the said project to use. Whether these sources are information sourced within or outside the organization, or they come in the form of software or algorithms, it is important that they are vouched for as accurate, up-to-date, complete, and valid that value can be derived from such data.
Collection Once the data source has been vouched for, the next step is to collect the data from that source, particularly the ones relevant to the organization. While the collection can be done manually, given the complexity that may be involved with the data, it is recommended that such work is to be done by automation, such as using software coded with specific programming language designed specifically for collecting relevant data from the source.
Wrangling Wrangling here pertains to the set of processes designed to transform raw data into a more usable format. Depending on the quality of the data in question, it may involve merging multiple datasets, identifying and filling gaps in data, deleting unnecessary or incorrect data, and “cleaning” and structuring data for future analysis. Data wrangling can be performed manually or via automation, depending on the size of the dataset involved.
Analysis After raw data has been wrangled, it can now be analyzed wherein users will be able to derive insightful or useful information from which actions or decisions can be made by the organization’s stakeholders. Such analysis can be diagnostic, descriptive, predictive, or prescriptive, depending on the organization’s needs, and usually involves some form of automation.
Storage Throughout all of the data life cycle stages, data must be stored in a manner that’s both secure and accessible by those authorized internally or externally by the organization that owns the data. Data can be stored on-site or in the cloud or other media, depending on the organization’s needs and data governance policies.
Why Understanding the Data Ecosystem Components Matter
Each component of the data ecosystem interacts with the other components and determines how these components process data on their end. What this means is that any of these components can introduce data integrity, privacy, and security threats if the organization does not conduct due diligence and does not monitor what is being done within each component.
By understanding how each component of the data ecosystem interacts with other components, your organization can better prepare for these kinds of challenges and identify opportunities for efficiency.
Comments