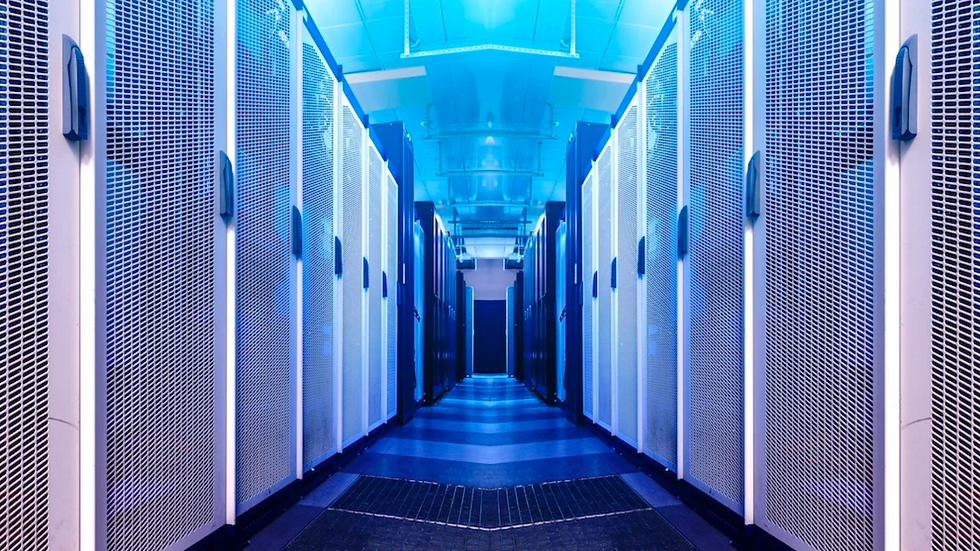
While it is important to ensure the data quality within the data warehouse, one challenge that has been often encountered in such efforts is the complicated process that is involved in cleansing the data. As such many organizations opt to sidestep this process in favor of accomplishing short-term goals, not realizing that these goals would end up for naught because they did not address the data quality issues in the first place.
Given these challenges, businesses need to take the following steps to ensure the quality of the data in their data warehouse:
1. Data Profiling
Data profiling is the process of analyzing and examining data to understand its characteristics, structure, and quality. This helps organizations better understand their data assets, identify any quality or consistency issues, and enhance the overall quality of their data, especially if they have large and complex data sets.
Data profiling tools can help organizations create a detailed inventory of their data assets and assess the appropriateness of the data for specific uses, as well as identify potential issues and better manage data quality.
2. Data Cleansing and Remediation
Once data profiling is completed, the next step is cleansing and remediation which involves identifying and correcting or removing any errors, inconsistencies, or missing information within the data. Cleansing involves removing or correcting any errors, inconsistencies, or inaccuracies in the data, while remediation involves addressing any underlying problems that have resulted in data quality issues which may involve updating data collection processes, improving data entry procedures, or identifying and correcting inconsistencies in different systems.
Ultimately, the goal of data cleansing and remediation is to ensure that the data is accurate, consistent, and reliable. This enables better decision-making, increased efficiency and productivity, and enhanced customer satisfaction.
3. Data Integration
Data integration refers to the process of combining and merging data (which should be cleansed and remediated by this point) from various sources into a unified and consistent format, creating a complete and accurate picture of an organization's data by extracting, transforming, and loading (ETL) data from different systems to create a central repository or data warehouse.
Moreover, data integration helps organizations unlock the full potential of their data by ensuring that it is trustworthy, accessible, and actionable. It is therefore critical for the organization to develop a clear data integration strategy that establishes the previous steps in place and ensure that their data is consistent, standardized, and conforms to best practices for data management.
4. Data Augmentation
The last step is to improve and update data through data augmentation, in which data is constantly verified and updated by cross-referencing internal and external databases. This is useful in situations involving customer data and product sales, where detailed information provides a more in-depth insight into the user data.
Through data augmentation, users can significantly reduce manual intervention to attain meaningful information for analytics and enhance data quality significantly.
Ensuring accurate and trustworthy data is a continuous endeavor that is a given in the dynamic nature that characterizes today’s business and technology environments. Indeed, it is a continuous endeavor that every organization must commit itself to. By prioritizing data quality at all times, organizations can empower themselves to make informed decisions, gain a competitive edge, and ultimately thrive in this competitive data-driven environment.
Comments