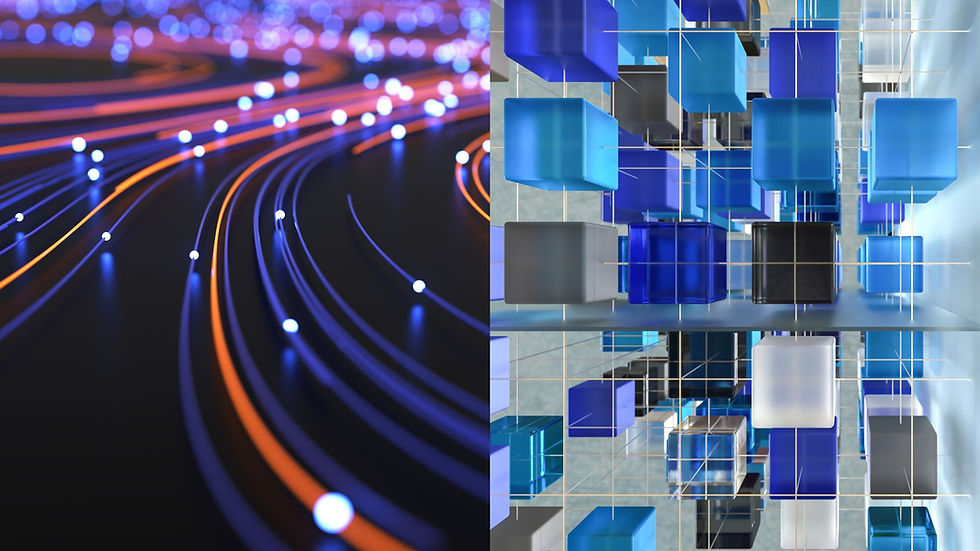
As large amounts of data are being accumulated each day online, the matter of storage and organization is becoming a concern, especially among businesses that have amassed so much data for their needs.
In addressing the need to store large data, two popular options have emerged, each with their own characteristics and advantages.
Data lake
The first data storage solution is the data lake, so-called because it is a vast pool of data that has yet to be organized into specific classifications or defined as to what purpose it is to be used for. While the lack of definition for the data in the data lake can make for a difficult data search, the data there is actually easily accessible since searching does not involve having to navigate hierarchies. For businesses, a data lake is also less costly to maintain.
Data warehouse
The second data storage solution is the data warehouse. Similar to the setup of the physical warehouse, data stored in the data warehouse is more organized, in which they are already structured, filtered, and have already been processed for a specific purpose by the business. The existing organization in place for the data may make it less strenuous in terms of search but accessibility to it can be more complicated as it involves specific policies and parameters. It also can be more costly to maintain due to the structures and definitions in place.
Despite the differences and the disparate nature of each, data lakes and data warehouses complement each other in a data workflow. Ingested company data will be stored in a data lake and if a specific business question comes up, a portion of the data deemed relevant is extracted from the lake, cleaned, and exported into a data warehouse.
Which solution is right for your business?
Data lake and data warehouse each has their own attributes that make them viable solutions depending on the organization’s needs. But how does the business determine the option that fits their needs? These will depend on the following criteria:
Purpose
Data lakes are used for the cost-effective storage of large amounts of data from many sources as such data is more flexible and scalable as they do not need to fit a specific schema. Structured data however requires it to be restricted to a schema, making it suitable for analytical work. Thus, such structured data are suited to be kept in a data warehouse as they are efficient for analyzing historical data.
Users
Since data in data warehouses are kept there for analysis, it is usually data analysts and business analysts who often work within data warehouses. It is also worth noting that data warehouses require a lower level of programming and data science knowledge to use.
Data lakes are set up and maintained by data engineers who integrate them into data pipelines. Data scientists work more closely with data lakes as they contain data of a wider and more current scope.
Tasks
Data engineers use data lakes to store incoming data and, most importantly, big data analytics. Big data analytics can be run on data lakes using services such as Apache Spark and Hadoop. This is especially true for deep learning, which requires scalability in the increasing amount of training data.
Data warehouses are typically set to read-only for analyst users, who are primarily reading and aggregating data for insights. Since data is already clean and archival, there is usually no need to insert or update data.
Size
For businesses that want to retain all data that might be relevant to them, data lakes are appropriate for their use. Data warehouses are much more selective as to what data is stored, making them appropriate for businesses with specific storage criteria set in place.
Data lakes and data warehouses provide specific solutions for different business needs and are instrumental in driving efficiency and growth for the business. The business should be able to determine which option would best suit their needs and workflows that will help them achieve their goals faster and more efficiently.
Comments